Generative AI Report – 9/19/2023
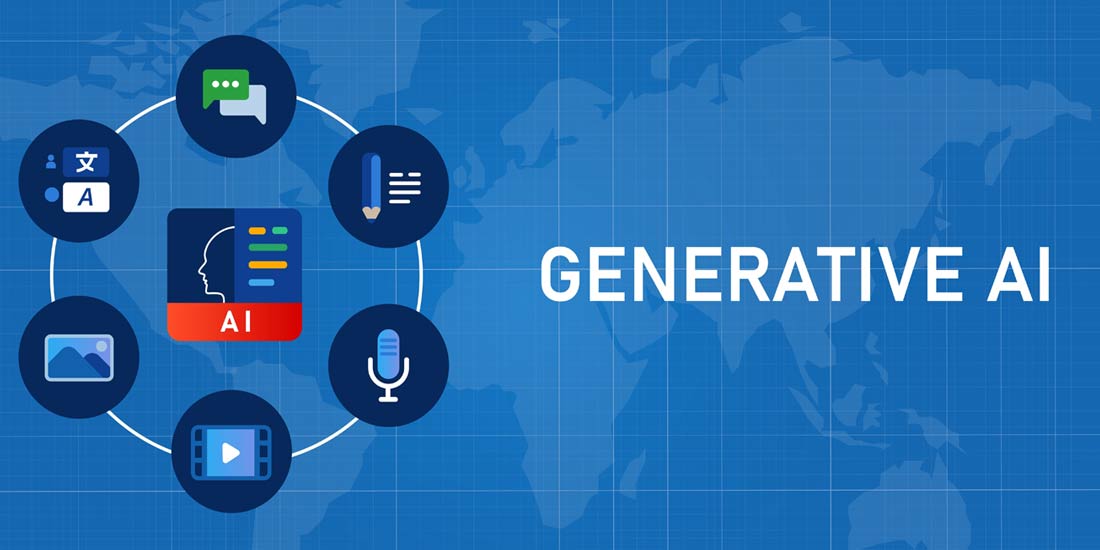
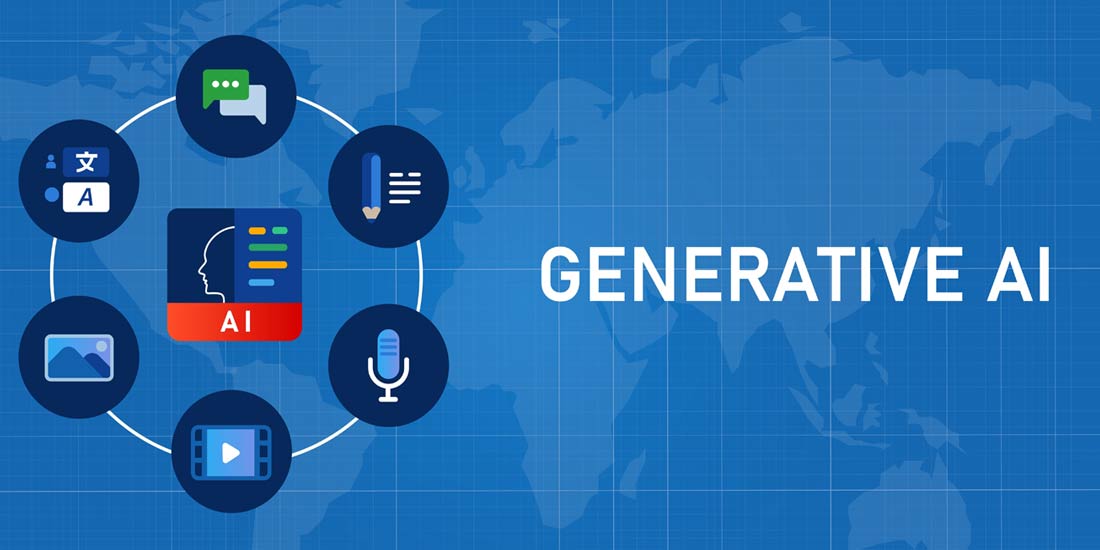
Welcome to the Generative AI Report round-up feature here on insideBIGDATA with a special focus on all the new applications and integrations tied to generative AI technologies. We’ve been receiving so many cool news items relating to applications and deployments centered on large language models (LLMs), we thought it would be a timely service for readers to start a new channel along these lines. The combination of a LLM, fine tuned on proprietary data equals an AI application, and this is what these innovative companies are creating. The field of AI is accelerating at such fast rate, we want to help our loyal global audience keep pace.
TruEra Launches “TruEra AI Observability,” First Full Lifecycle AI Observability Solution Covering Both Generative and Traditional AI
TruEra launched TruEra AI Observability, a full-lifecycle AI observability solution providing monitoring, debugging, and testing for ML models in a single SaaS offering. TruEra AI Observability now covers both generative and traditional (discriminative) ML models, meeting customer needs for observability across their full portfolio of AI applications, as interest in developing and monitoring LLM-based apps is accelerating.
Initial development of LLM-based applications is dramatically increasing since the launch of ChatGPT. However, LLM-based applications have well-known risks for hallucinations, toxicity and bias. TruEra AI Observability offers new capabilities for testing and tracking LLM apps in development and in live use, so that risks are minimized while acceleratingLLM app development. The product capabilities were informed by the traction of TruLens – TruEra’s open source library for evaluating LLM applications.
“TruEra’s initial success was driven by customers in banking, insurance, and other financial services, whose high security requirements were well met by existing TruEra on-prem solutions,” said TruEra Co-founder, President and Chief Scientist Anupam Datta. “Now, with TruEra AI Observability, we are bringing ML monitoring, debugging, and testing to a broader range of organizations, who prefer the rapid deployment, scalability, and flexibility of SaaS. We were excited to see hundreds of users sign up in the early beta period, while thousands have engaged with our hands-on educational offerings and community. The solution brings incredible monitoring and testing capabilities to everyone developing machine learning models and LLM applications.”
Vianai Introduces Powerful Open-Source Toolkit to Verify Accuracy of LLM-Generated Responses
Vianai Systems, a leader in human-centered AI (H+AI) for the enterprise, announced the release of veryLLM, an open-source toolkit that enables reliable, transparent and transformative AI systems for enterprises. The veryLLM toolkit empowers developers and data scientists to build a universally needed transparency layer into Large Language Models (LLMs), to evaluate the accuracy and authenticity of AI-generated responses — addressing a critical challenge that has prevented many enterprises from deploying LLMs due to the risks of false responses.
AI hallucinations, in which LLMs create false, offensive or otherwise inaccurate or unethical responses raise particularly challenging issues for enterprises as the risks of financial, reputational, legal and/or ethical consequences are extremely high. The AI hallucination problem left unaddressed by LLM providers has continued to plague the industry and hinder adoption, with many enterprises simply unwilling to bring the risks of hallucinations into their mission-critical enterprise systems. Vianai is releasing the veryLLM toolkit (under the Apache 2.0 open-source license) to make this capability available for anyone to use, to build trust and to drive adoption of AI systems.
The veryLLM toolkit introduces a foundational ability to understand the basis of every sentence generated by an LLM via several built-in functions. These functions are designed to classify statements into distinct categories using context pools that the LLMs are trained on (e.g., Wikipedia, Common Crawl, Books3 and others), with the introductory release of veryLLM based on a subset of Wikipedia articles. Given that most publicly disclosed LLM training datasets include Wikipedia, this approach provides a robust foundation for the veryLLM verification process. Developers can use veryLLM in any application that leverages LLMs, to provide transparency on AI generated responses. The veryLLM functions are designed to be modular, extensible, and work alongside any LLM, providing support for existing and future language models.
“AI hallucinations pose serious risks for enterprises, holding back their adoption of AI. As a student of AI for many years, it is also just well-known that we cannot allow these powerful systems to be opaque about the basis of their outputs, and we need to urgently solve this. Our veryLLM library is a small first step to bring transparency and confidence to the outputs of any LLM – transparency that any developer, data scientist or LLM provider can use in their AI applications,” said Dr. Vishal Sikka, Founder and CEO of Vianai Systems and advisor to Stanford University’s Center for Human-Centered Artificial Intelligence. “We are excited to bring these capabilities, and many other anti-hallucination techniques, to enterprises worldwide, and I believe this is why we are seeing unprecedented adoption of our solutions.”
Pinecone working with AWS to solve Generative AI hallucination challenges
Pinecone, the vector database company providing long-term memory for artificial intelligence (AI), announced an integration with Amazon Bedrock, a fully managed service from Amazon Web Services (AWS) for building GenAI applications. The announcement means customers can now drastically reduce hallucinations and accelerate the go-to-market of Generative AI (GenAI) applications such as chatbots, assistants, and agents.
The Pinecone vector database is a key component of the AI tech stack, helping companies solve one of the biggest challenges in deploying GenAI solutions — hallucinations — by allowing them to store, search, and find the most relevant and up-to-date information from company data and send that context to Large Language Models (LLMs) with every query. This workflow is called Retrieval Augmented Generation (RAG), and with Pinecone, it aids in providing relevant, accurate, and fast responses from search or GenAI applications to end users.
With Amazon Bedrock, the serverless platform lets users select and customize the right models for their needs, then effortlessly integrate and deploy them using popular AWS services such as Amazon SageMaker.
Pinecone’s integration with Amazon Bedrock allows developers to quickly and effortlessly build streamlined, factual GenAI applications that combine Pinecone’s ease of use, performance, cost-efficiency, and scalability with their LLM of choice. Pinecone’s enterprise-grade security and its availability on the AWS Marketplace allow developers in enterprises to bring these GenAI solutions to market significantly faster.
“We’ve already seen a large number of AWS customers adopting Pinecone,” said Edo Liberty, Founder & CEO of Pinecone. “This integration opens the doors to even more developers who need to ship reliable and scalable GenAI applications… yesterday.”
Sign up for the free insideBIGDATA newsletter.
Join us on Twitter: https://twitter.com/InsideBigData1
Join us on LinkedIn: https://www.linkedin.com/company/insidebigdata/
Join us on Facebook: https://www.facebook.com/insideBIGDATANOW