The Importance of Protecting AI Models
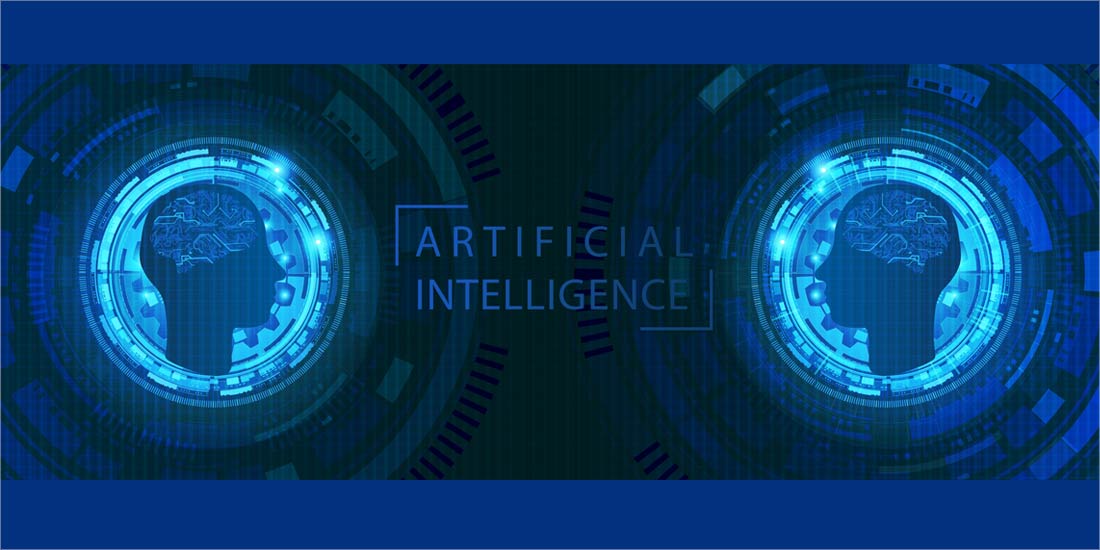
Over the last decade, we’ve seen the rapid advancement of security threats deeper into the technology stack. What started within software and applications has moved to operating systems and middleware, on to firmware and hardware, and now through pathways driven by Artificial Intelligence (AI) tools and technologies. It’s no secret that AI is disrupting the technology landscape; keeping data and models safe is becoming critically important for organizations, corporations, and our society at large.
Today, a wide variety of organizations are leveraging AI to analyze and make use of massive quantities of data. In fact, Bloomberg Intelligence predicts the AI market will grow to $1.3 trillion over the next 10 years. But according to Forrester Research, 86% of organizations are extremely concerned or concerned about their organization’s AI model security.
That number is not surprising given the broad range of malicious attacks being directed at AI models, including training-data poisoning, AI model theft, adversarial sampling, and more. To date, the MITRE ATLAS™ (Adversarial Threat Landscape for Artificial-Intelligence Systems) framework has cataloged more than 60 ways to attack an AI model.
As a result, governments around the world are issuing new regulations to help keep AI deployments secure, trustworthy, and private. These include the European Union’s AI Act and the U.S. Executive Order on the Safe, Secure Artificial Intelligence. When these new regulations are combined with existing regulations like GDPR and HIPAA, they present an even more complex cybersecurity and privacy regulatory landscape that enterprises must navigate when designing and operating AI systems.
Throughout their lifecycles, leaving AI models and their data training sets unmanaged, unmonitored, and unprotected can put an organization at risk for data theft, fines, and more. After all, the models are often the definition of critical intellectual property, and the data is often sensitive, private, or regulated. AI deployments involve a pipeline of activities from initial data acquisition to the final results. At each stage, an adversary could take action that manipulates the model’s behavior or steals valuable intellectual property. Alternatively, poorly managed data practices could lead to costly compliance violations or a data breach that must be disclosed to customers.
Given there is a need to protect these models and their data while aligning to compliance requirements, how is it being done? One available tool is Confidential AI. Confidential AI is the deployment of AI systems inside Trusted Execution Environments (TEE) to protect sensitive data and valuable AI models while they are actively in-use. By design, TEEs prevent AI models and data from being seen in the clear by any application or user that is not authorized. And all elements within a TEE (including the TEE itself) should be attested by any operator neutral party before those keys are sent for learning and inference inside the TEE. These attributes provide the owner of the data or model with enhanced control over their IP and data (since they have the ability to enforce attestation and customer policy adherence before releasing the keys).
Encryption for data at-rest in storage, or in-transit on a network, is an established practice. But protecting data that is actively in use has been a challenge. Confidential Computing helps solve that problem with hardware-based protections for data in the CPU, GPU, and memory. Now Confidential AI takes modern AI techniques, including Machine Learning and Deep Learning, and overlays them with traditional Confidential Computing technology.
What are some use cases? Let’s look at three. But keep in mind that Confidential AI use cases can apply at any stage in the AI pipeline, from data ingestion and training to inference and results interface.
The first is collaborative AI. When analyzing data from multiple parties, each party in the collaboration will contribute their encrypted data sets, providing protections so each party cannot see the data from the other party. Using a Confidential Computing enabled Data Clean Room, which is secured by a Trusted Execution Environment, enables organizations to collaborate on data analytics projects while maintaining the privacy and security of the data and the models. Data Clean Rooms are becoming increasingly important in the context of AI and ML. This type of multiparty data analytics and AI/ML can enable organizations to collaborate on data driven AI research.
The next example is Federated Learning, a form of collaborative AI. In this case, assume the data is too big, sensitive, or regulated to move off-premises. Instead, the compute is moved to the data. A node configured with a TEE and the model are deployed at each party’s location. The data is used to train the model locally, while any proprietary model IP is protected inside the TEE. The updated weights are encrypted and then communicated to a master model in the cloud, where they are merged with weights from the other parties.
The last example will increasingly be deployed as organizations use Large Language Models (LLMs) to process sensitive queries or perform tasks using confidential data. In this model, the model query engine is protected inside a TEE. Queries are encrypted during transfer to a private LLM, also deployed in a TEE. The results from the model are encrypted and transferred back to the requestor. The query or its results are designed to be unavailable in plaintext outside a TEE, providing end-to-end protection.
As businesses of all sizes continue to adopt AI, it’s clear they’ll need to protect their data, IP, and corporate integrity. Doing so requires that security products integrate both data science models and frameworks, as well as the connected applications that operate in the public-facing “real world.” A comprehensive and proactive security and compliance posture for AI should enable an organization to devise, develop, and deploy machine learning models from day one in a secure environment, with real-time awareness that’s easy to access, understand, and act upon.
About the Author
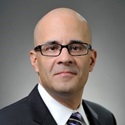
Rick Echevarria, Vice President, Security Center of Excellence, Intel
Sign up for the free insideBIGDATA newsletter.
Join us on Twitter: https://twitter.com/InsideBigData1
Join us on LinkedIn: https://www.linkedin.com/company/insidebigdata/
Join us on Facebook: https://www.facebook.com/insideBIGDATANOW